To be a real data-driven marketer you have to look past ‘data for knowing’ and understand ‘data for doing’.
Data has become the cornerstone of effective marketing strategies. As a marketing director, it’s crucial to understand where your organisation stands on the analytics maturity curve. This is especially important if the data strategy of your business is held by a non-marketing department. You can motivate for the future state of your marketing initiatives by knowing what is possible once you breach the traditional data warehouse and visualisation tools level that most organisations are stuck at.
In this guide, we’ll explore the different stages of analytics maturity, identify common pitfalls, and outline some use cases at each stage.
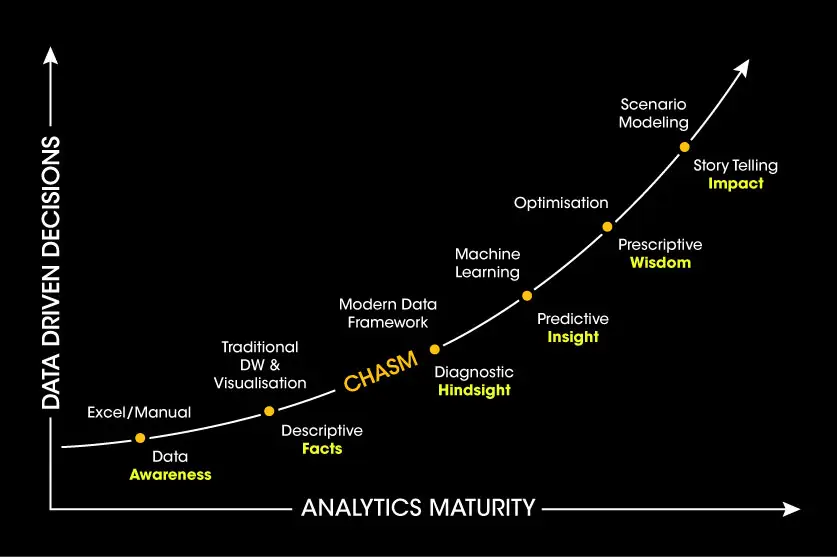
Where Most Are: Data Awareness and Descriptive Facts
At the initial stage of the analytics maturity curve, many organisations operate with basic data awareness. This often involves using tools like Excel spreadsheets or manual processes to collect and analyse data. While these methods can provide valuable insights, they are limited in their ability to handle large datasets and complex analysis.
Use case: Consider a small e-commerce business that relies on manual data entry to track sales and customer information. They use Excel spreadsheets and google analytics to organize data such as customer demographics, purchase history, and website traffic. While this approach gives them some understanding of their customers’ behavior, it lacks the sophistication to uncover deeper insights or predict future trends.
As organizations progress, they typically adopt more sophisticated tools such as traditional data warehouses and visualisation platforms. These tools enable marketers to gain descriptive facts about their audience, such as demographic information, purchase history, and website engagement metrics. While these insights are valuable for understanding past performance, they only scratch the surface of what predictive analytics can offer.
Use case: A retail chain utilises a traditional data warehouse to store and analyse vast amounts of customer data. They use visualisation platforms such as – PowerBi or Tableau – to create reports and dashboards that provide descriptive insights into customer behavior, such as purchase patterns and product preferences. While this helps them understand historical trends, they struggle to translate these insights into actionable strategies for the future.
This is the ‘data for knowing’ phase and where the majority of companies currently sit. The process tends to stall here because people believe that they can ‘see’ all the data effectively and they are best placed to make decisions based on it. The future state is understanding that there are some things that can now be done better by machine learning as it can see significantly more than it’s human handlers will ever be able to.
Current Gap: The Leap to a Modern Data Framework
The next step in the analytics maturity journey is bridging the gap between descriptive insights and predictive capabilities.
This requires adopting a modern data framework that goes beyond traditional data warehouses to provide diagnostic hindsight and machine learning capabilities. A modern data framework is characterised by its ability to handle diverse data types, including structured, semi-structured, and unstructured data, and its flexibility to scale and adapt to changing business needs. This means that you can throw any data type at it and it can manage it, including images, PDF’s and audio. This framework leverages technologies such as data lakes, distributed computing, and cloud platforms to store and process large volumes of data efficiently.
In the context of machine learning capabilities, a modern data framework enables organisations to leverage advanced analytics techniques to uncover hidden patterns and insights within their data. Unlike traditional analytics approaches that focus on descriptive and diagnostic analysis (i.e., what happened and why it happened), machine learning enables predictive and prescriptive analysis (i.e., what will happen and how to make it happen).
Use case: An Insurance provider recognises the limitations of their current data infrastructure. They begin the process of centralising data from sales and marketing, digital platforms, loyalty apps, health screenings, medical records, Product Administration Systems and CRM’s. They invest in a modern data framework that incorporates machine learning algorithms to analyse user behavior and predict which products they are likely to need next. This allows them to personalise recommendations in marketing and improve customer satisfaction, ultimately leading to increased retention and revenue.
Machine learning plays a pivotal role in this leap towards a modern data framework. By leveraging machine learning algorithms, organizations can unlock hidden patterns and correlations within their data, enabling them to make more accurate predictions and informed decisions.
Use case: A fashion retailer uses machine learning algorithms to analyse historical sales data and identify trends in customer preferences. By understanding which products are likely to be popular in the upcoming seasond, they can optimise their inventory management and marketing strategies to meet consumer demand effectively.
Incorporating machine learning into the data framework also empowers marketers to automate repetitive tasks and processes, allowing them to focus on more strategic initiatives. This is the stage of ‘data for doing’. Rather than rely on humans to action initiatives based on
Use case: A marketing department automates the process of segmenting their customer base using machine learning algorithms. This allows them to create targeted marketing campaigns tailored to specific customer segments, resulting in higher engagement and conversion rates. These segments are dynamic and update continuously to automate the sending of communications to the right customer, at the right time.
Future State: Building Towards Prescriptive Wisdom
As organisations continue to mature in their analytics journey, the ultimate goal is to reach a state of prescriptive wisdom.
Prescriptive wisdom involves leveraging advanced analytics techniques to optimise marketing efforts and drive business outcomes. This can include building on existing data depositories to add data that is not even generated by the business, eg geo-spatial data or economic indicators,
Use case: A global technology company leverages advanced analytics to optimize their digital marketing campaigns. By analysing data from various sources, including social media, website traffic, weather patterns and customer interactions, they gain prescriptive insights into the most effective marketing tactics. This allows them to allocate resources more efficiently, target the right audience segments, and tailor messaging to individual preferences, resulting in higher ROI and customer engagement.
More importantly, advanced analytics enables marketers to engage in scenario building, allowing them to simulate different outcomes based on various factors. This “storytelling impact” approach empowers marketing directors to anticipate market trends, identify potential risks, and capitalise on opportunities before they arise.
Use case: A consumer goods company uses scenario building to anticipate the impact of external factors, such as changes in consumer preferences or economic conditions, on their sales forecasts. By analysing historical data and running simulations, they can develop contingency plans and adjust their marketing strategies accordingly to mitigate risks and maximise profitability.
Understanding where your organisation stands on the analytics maturity curve is crucial for unlocking the full potential of predictive analytics and scenario building in marketing. By recognising the current state, bridging the gap with a modern data framework, and building towards prescriptive wisdom, marketing directors can drive better decision-making, optimise marketing efforts, and ultimately achieve business success in today’s data-driven landscape.